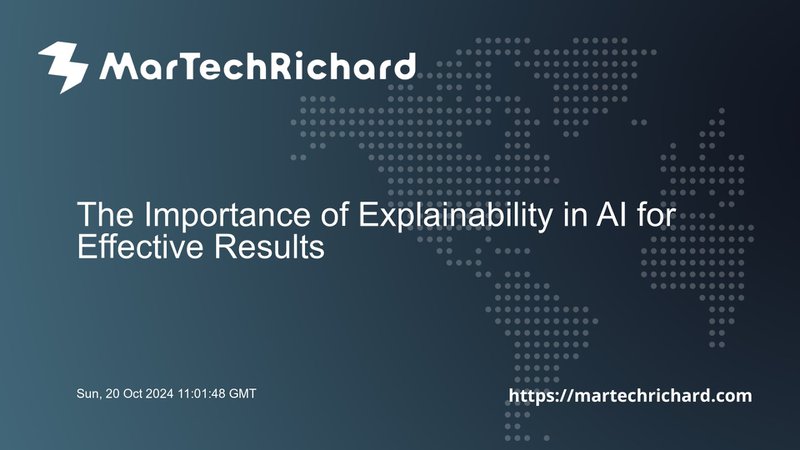
Why Explainability Matters in AI
Summary
The article "Why Explainability Matters in AI" on Towards Data Science highlights the critical importance of explainability in artificial intelligence. Explainability refers to the ability of an AI system to provide transparent and understandable insights into its decision-making processes. This transparency is essential for building trust and ensuring the ethical use of AI systems. The article emphasizes that as AI becomes increasingly integrated into various aspects of life, including business operations and critical decision-making processes, it is crucial to understand how these models derive their conclusions.
Background Information
Explainability in AI is not just about making the technology more understandable; it's about ensuring that the decisions made by AI systems are fair, secure, and transparent. This involves understanding the data used to train the models and the algorithms employed. Advanced machine learning techniques like deep learning and neural networks often operate as "black boxes," making it challenging for humans to comprehend their inner workings. However, developing tools and processes that can explain these complex models is vital for their adoption and trustworthiness.
Key Points from the Article
- Importance of Explainability:Explainability is crucial for building trust among users and stakeholders. It ensures that AI systems are transparent and fair in their decision-making processes.
Companies that prioritize explainability are more likely to see significant bottom-line returns from their AI investments. - Challenges in Explainability:Advanced machine learning models are inherently difficult to understand due to their complex operations.
The solution lies not in simply conveying how a system works but in creating tools and processes that can help even deep experts understand and explain the outcomes. - Benefits of Explainable AI:Techniques that enable explainability can increase productivity by quickly revealing errors or areas for improvement.
Explainable AI helps in building trust and adoption by providing clear insights into how decisions are made. - Implementing Explainability in Organizations:Organizations should include explainability as a key principle within their responsible AI guidelines.
Establishing an AI governance committee can set standards and guidelines for AI development teams, ensuring that explainability is integrated into all stages of model development.
Additional Insights
- Trade-offs Between Explainability and Accuracy:While simplifying an AI model's mechanics might improve user trust, it can sometimes make the model less accurate. Organizations must weigh these trade-offs, considering regulatory requirements and potential impacts on performance.
- Investment in Explainability Technology:Investing in appropriate tools for meeting the needs identified by development teams is essential. Advanced tooling may provide robust explanations in contexts where accuracy might otherwise be sacrificed.
- Legal and Ethical Considerations:Legal requirements such as the EU AI Act mandate human oversight of model predictions and prohibit discriminatory decision-making based on protected characteristics. Ensuring explainability is crucial for compliance with these regulations.
Discussion:
Q1: How can organizations balance the need for explainability with the potential trade-offs in model accuracy?
A1: Balancing Explainability and Model Accuracy in Organizations
Organizations face the challenge of balancing the need for explainability in AI models with the potential trade-offs in model accuracy. This balance is crucial, especially in high-stakes environments such as healthcare, finance, and legal sectors.
Importance of Explainability
Explainability refers to the clarity with which an AI model's decisions can be understood by humans. It is particularly important in scenarios where:
- Trust and Accountability: Stakeholders need to trust AI decisions, especially when they impact lives or finances. For instance, a cancer diagnosis based on an AI model requires transparency to ensure that medical professionals can validate the results.
- Error Reduction: Explanations can help identify and rectify errors in AI predictions. For example, if an AI model flags an invoice amount, understanding how it arrived at that figure can prevent costly mistakes.
- Regulatory Compliance: Many industries are subject to regulations that require transparency in decision-making processes. A clear explanation of how conclusions were reached can demonstrate compliance and reduce legal risks.
Trade-offs with Accuracy
While explainability is vital, it can sometimes come at the cost of accuracy:
- Complexity of Models: Highly accurate models, such as deep learning networks, often operate as "black boxes." Their internal workings are not easily interpretable, which can lead to a lack of explainability.
- Focus on Performance Over Clarity: In some cases, organizations may prioritize improving model accuracy over providing explanations. For example, in medical imaging, achieving a higher diagnostic accuracy might be deemed more critical than understanding the reasoning behind a specific diagnosis.
Strategies for Balancing Both Needs
Organizations can adopt several strategies to strike a balance between explainability and accuracy:
- Hybrid Approaches: Use a combination of interpretable models (like decision trees) alongside complex models. This allows organizations to leverage the strengths of both while maintaining some level of transparency.
- Explainable AI Techniques: Implement techniques such as LIME (Local Interpretable Model-agnostic Explanations) or SHAP (SHapley Additive exPlanations) that provide insights into model predictions without sacrificing accuracy significantly.
- Human-in-the-loop Systems: Incorporating human oversight can enhance both explainability and accuracy. By allowing human analysts to review and validate AI outputs, organizations can ensure that decisions are both reliable and transparent.
- Iterative Development: Continuously refine models based on feedback from users regarding their need for explanations. This iterative process helps align model performance with user expectations for clarity.
In conclusion, while there are inherent trade-offs between explainability and accuracy in AI models, organizations can employ various strategies to mitigate these challenges. By prioritizing both aspects appropriately, they can enhance trust and reliability in their AI systems while ensuring compliance with regulatory standards.
Q2.What role does explainability play in ensuring the ethical use of AI systems?
A2: The Role of Explainability in Ensuring Ethical Use of AI Systems
Explainability is a crucial aspect of ensuring the ethical use of artificial intelligence (AI) systems. It serves multiple functions that contribute to the responsible deployment and governance of AI technologies.
Building Trust and Accountability
- Transparency: Explainability enhances transparency by allowing stakeholders to understand how AI systems make decisions. This understanding is essential for building trust among users, regulators, and the public. When individuals can see the rationale behind AI decisions, they are more likely to accept and trust those outcomes.
- Accountability: By providing clear explanations for decisions made by AI systems, organizations can better attribute responsibility for those decisions. This accountability is vital in cases where AI systems impact individual rights or public safety, ensuring that there are mechanisms in place to address any adverse outcomes.
Mitigating Risks and Bias
- Identifying Bias: Explainable AI helps organizations uncover biases inherent in their algorithms. Understanding how decisions are made allows for the identification and correction of discriminatory practices that may arise from biased training data or flawed model assumptions.
- Regulatory Compliance: Many jurisdictions are implementing regulations that require organizations to explain the decisions made by their AI systems. For instance, the California Department of Insurance mandates explanations for adverse actions based on complex algorithms. Compliance with such regulations not only protects organizations from legal repercussions but also promotes ethical standards in AI deployment.
Enhancing User Engagement and Understanding
- User Empowerment: When users understand how an AI system arrives at its conclusions, they can engage more effectively with the technology. This empowerment leads to better decision-making and fosters a collaborative environment between human users and AI systems.
- Informed Decision-Making: Explainability enables users to make informed choices based on AI recommendations. For example, in healthcare, understanding why an AI model suggests a particular treatment can help medical professionals make better decisions for their patients.
In conclusion, explainability plays a pivotal role in ensuring the ethical use of AI systems by fostering trust, accountability, risk mitigation, and user engagement. As AI technologies continue to evolve, prioritizing explainability will be essential for promoting ethical standards and responsible practices in their deployment.
Q3. What technological advancements are currently being explored to enhance explainability in complex AI models?
A3:Technological Advancements in Enhancing Explainability in AI Models
As the demand for transparency in AI systems grows, various technological advancements are being explored to enhance explainability in complex AI models. These innovations aim to address the "black box" problem and improve user trust and understanding of AI decision-making processes.
Key Techniques for Explainability
- Local Interpretable Model-agnostic Explanations (LIME): LIME provides insights into individual predictions by creating a local surrogate model around a specific instance. It perturbs the input data slightly and observes how the predictions change, allowing users to understand which features influenced the output most significantly.
- SHapley Additive exPlanations (SHAP): SHAP quantifies the contribution of each feature to a model's prediction using cooperative game theory principles. This method helps illustrate how different features impact the final decision, making it easier for users to grasp the model's behavior.
- CXPlain: This newer method estimates feature importance while addressing some limitations of LIME and SHAP, such as high computational costs. CXPlain trains a standalone model based on errors from the original black-box model, providing insights into feature contributions along with confidence estimates for predictions.
Global and Local Approaches
- Global Surrogate Models: These models aim to represent the overall decision logic of complex AI systems by training simpler, interpretable models (like linear regression) on the input-output pairs from the black box. While they can provide a general understanding, their effectiveness depends on the quality of the underlying data.
- Visualization Techniques: Visual tools play a significant role in explainability. Techniques like decision boundaries and activation maps help users visualize how models classify data or which parts of an input are most influential in making decisions. Such visualizations can simplify complex concepts and enhance user comprehension.
Emerging Technologies
- Knowledge Graphs: Companies are integrating diverse data formats into knowledge graphs to enhance transparency in AI systems. This approach allows for better contextualization of data and improves reliability in areas like medical diagnoses by making relationships between different data points clearer.
- Explainable AI (XAI) Frameworks: Organizations are developing comprehensive frameworks that incorporate explainability as a core principle in AI development. This includes establishing governance committees to set standards and guidelines for creating interpretable AI systems, ensuring ethical considerations are integrated from the outset.
- Feedback Mechanisms: Some XAI systems now include features that allow users to provide feedback on the helpfulness of explanations. This iterative process can improve the quality of explanations over time and adapt them to user needs.
In conclusion, advancements in explainability are essential for fostering trust and accountability in AI systems. By employing techniques like LIME, SHAP, CXPlain, and utilizing visualization methods alongside emerging technologies like knowledge graphs, organizations can enhance understanding and ensure ethical use of AI across various sectors.
Contact us
If you're interested in learning more about how to implement explainability in your AI systems, contact us via email at [email protected] for further inquiry. You can also reach out to us via LinkedIn message and subscribe to our LinkedIn page and newsletters via LinkedIn Page.
Source: Towards Data Science – Why Explainability Matters in AI