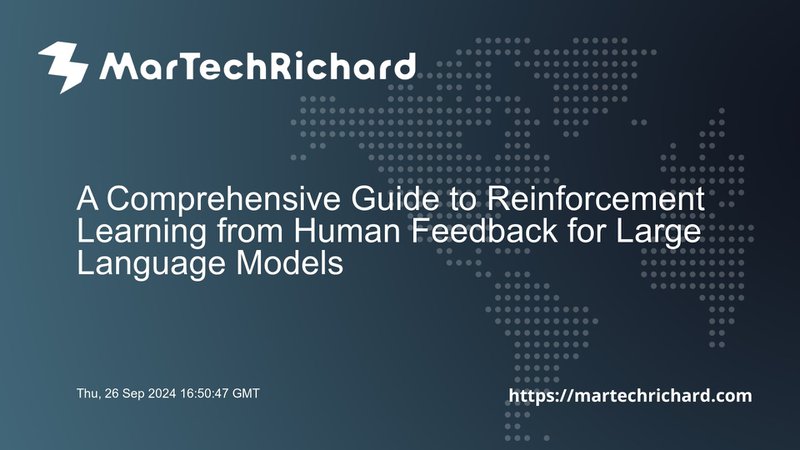
Reinforcement Learning from Human Feedback (RLHF) for LLMs: A Game-Changer in AI
Reinforcement Learning from Human Feedback (RLHF) has revolutionized the training of Large Language Models (LLMs) by integrating human guidance into the reinforcement learning process. This technique is particularly notable for its application in models like ChatGPT, which has significantly improved the accuracy and coherence of generated text. In this blog post, we'll delve into the key points of RLHF, its benefits, and potential future developments.
Summary of RLHF
RLHF combines reinforcement learning techniques with human feedback to train AI agents, particularly LLMs, to generate text that is both engaging and factually accurate. The process involves three primary phases:
- Pretraining a Language Model: The initial phase involves selecting a pre-trained language model that responds well to diverse instructions. This model serves as the foundation for further fine-tuning.
- Reward Model Training: In the second phase, a reward model is trained using human feedback. This model predicts how humans will rate the quality of text generated by the LLM, providing a scalar reward that guides the reinforcement learning process.
- Fine-Tuning with Reinforcement Learning: The final phase involves fine-tuning the LLM using the reward model. The LLM is rewarded for generating text that is highly rated by the reward model, leading to continuous improvement through iterative human feedback and reinforcement learning.
Additional Insights
RLHF's success lies in its ability to align AI outputs with human values and preferences. For instance, models trained with RLHF can reject questions that are outside the scope of the request, ensuring that generated content is safe and respectful. This technique is not limited to NLP; it has also been applied in other areas like video game bots, making them more human-like players.
One of the significant benefits of RLHF is its adaptability. By fine-tuning models with various prompts and human feedback, LLMs can perform a wide range of tasks efficiently. This adaptability brings us closer to achieving general-purpose AI. Additionally, RLHF's iterative process ensures continuous improvement as new human feedback is integrated into the model's training.
Future Developments and Potential Impact
Despite its impressive results, RLHF is still a relatively new technique with much room for improvement. Future research aims to make LLMs more efficient, reduce their environmental footprint, and address some of the risks associated with LLMs. For instance, incorporating reinforcement learning from AI feedback (RLAIF) could further enhance model performance by leveraging AI-generated feedback.
The impact of RLHF on businesses and industries is substantial. By ensuring that AI-generated content is accurate, helpful, and respectful, companies can leverage LLMs for customer service, content creation, and more. For example, chatbots trained with RLHF can provide more natural-sounding responses that better address user queries, enhancing customer satisfaction.
Discussion Questions or Prompts
- How can businesses leverage RLHF to improve their customer service chatbots?Discuss the potential benefits and challenges of integrating RLHF into existing chatbot systems.
- What are the limitations of RLHF, and how can they be addressed in future developments?Explore the current limitations of RLHF and propose potential solutions for overcoming them.
- How does RLHF align with human values and preferences, and what are the implications for ethical AI development?Analyze how RLHF ensures that AI-generated content aligns with human values and discuss the ethical implications of this alignment.
Contact Us for Further Inquiry
If you're interested in learning more about how RLHF can be applied in your business or industry, feel free to contact us via WhatsApp at go.martechrichard.com/whatsapp or reach out to us via LinkedIn message. Don't forget to subscribe to our LinkedIn page and newsletters for the latest updates on AI and martech trends.
Source URL: Towards Data Science – Reinforcement Learning from Human Feedback (RLHF) for LLMs