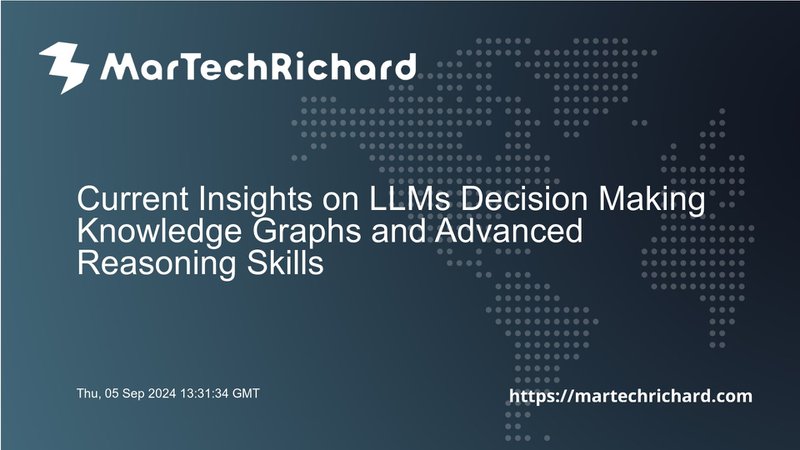
The Latest on LLMs: Decision-Making, Knowledge Graphs, Reasoning Skills, and More
Summary
The field of Large Language Models (LLMs) is rapidly evolving, with new models and research emerging daily. This article from Towards Data Science highlights the current state of LLMs, focusing on their decision-making capabilities, integration with knowledge graphs, and reasoning skills. LLMs have revolutionized natural language processing by enabling machines to understand and generate human language with high accuracy. However, they often struggle with maintaining context, handling ambiguous queries, and avoiding hallucinations.
Knowledge graphs have emerged as a powerful tool to enhance the contextual understanding and accuracy of LLMs. By structuring information in a way that resolves ambiguities and ensures accuracy, knowledge graphs significantly boost LLM performance. For instance, LLMs powered by knowledge graphs can achieve an astonishing accuracy increase from 16% to 72% for question answering over enterprise SQL databases. This synergy between LLMs and knowledge graphs has the potential to revolutionize industries such as healthcare, finance, education, and customer service by providing accurate and contextually relevant information.
Additional Insights
- Enhancing Reasoning Capabilities: The integration of knowledge graphs with LLMs is crucial for enhancing their reasoning capabilities. Knowledge graphs provide structured context that helps LLMs infer new implicit knowledge by reasoning over what is already known. This is particularly important for tasks like question answering and recommendation systems.
- Limitations of LLMs: While LLMs display strong few-shot learning abilities and can perform well on question answering and reasoning tasks, they lack grounded knowledge and reasoning capabilities. Their knowledge is confined to what can be learned from modeling word co-occurrence statistics on text corpora alone. This limitation can be addressed by integrating symbolic logic and intricate relationships from humans into neural networks, enhancing their pattern recognition prowess.
- Future Developments: Recent research suggests that LLMs are more suited as inference assistants rather than few-shot information extractors. This is evident in their performance in tasks related to knowledge graph construction and reasoning. For example, GPT-4 exhibits good performance in entity and relation extraction but excels further in reasoning tasks, surpassing fine-tuned models in certain cases.
Discussion Questions or Prompts
- How can knowledge graphs be effectively integrated with LLMs to enhance their decision-making capabilities?
- What are the potential applications of LLMs in industries such as healthcare and finance, and how can knowledge graphs support these applications?
- What are the limitations of current LLMs, and how can they be addressed through the integration of knowledge graphs and other AI techniques?
Lead Generation
If you're interested in learning more about how to leverage LLMs and knowledge graphs for your business, feel free to contact us via WhatsApp at go.martechrichard.com/whatsapp or reach out to us on LinkedIn. Subscribe to our LinkedIn page and newsletters via LinkedIn for the latest insights and updates on AI and martech.